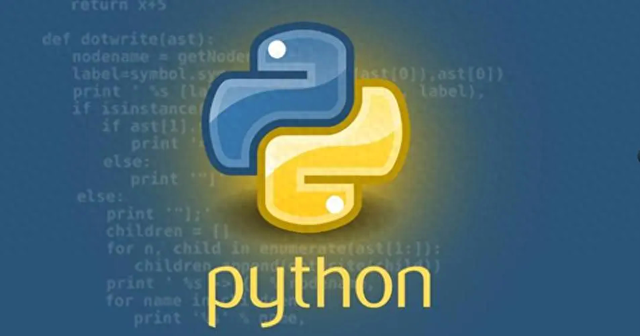
N-gram是自然语言处理中常用的技术,它可以用于文本生成、语言模型训练等任务。本文将介绍什么是n-gram,如何在Python中实现n-gram文本生成,并提供丰富的示例代码来帮助大家更好地理解和应用这一技术。
什么是N-gram?N-gram是自然语言处理中的一种文本建模技术,用于对文本数据进行分析和生成。它是一种基于n个连续词语或字符的序列模型,其中n表示n-gram的大小。通常,n的取值为1、2、3等。
Unigram(1-gram):一个单词或一个字符为一个单位。例如,"I", "love", "Python"。Bigram(2-gram):两个相邻的单词或字符为一个单位。例如,"I love", "love Python"。Trigram(3-gram):三个相邻的单词或字符为一个单位。例如,"I love Python"。N-gram模型通过分析文本中不同n-gram的出现频率,可以用于文本分类、文本生成、语言模型等任务。
实现N-gram文本生成下面将演示如何在Python中实现N-gram文本生成。将使用一个简单的示例来说明这一过程。
1 准备文本数据首先,需要准备一些文本数据,这将作为训练数据。这里使用了莎士比亚的一些文本作为示例数据,可以使用自己的文本数据。
text = """To be or not to be, that is the question;Whether 'tis nobler in the mind to sufferThe slings and arrows of outrageous fortune,Or to take arms against a sea of troublesAnd by opposing end them. To die—to sleep,No more; and by a sleep to say we endThe heart-ache and the thousand natural shocksThat flesh is heir to, 'tis a consummationDevoutly to be wish'd. To die, to sleep;To sleep, perchance to dream—ay, there's the rub,For in that sleep of death what dreams may come,When we have shuffled off this mortal coil,Must give us pause—there's the respectThat makes calamity of so long life;The oppressor's wrong, the proud man's contumely,The pangs of despis'd love, the law's delay,The insolence of office, and the spurnsThat patient merit of the unworthy takes,When he himself might his quietus makeWith a bare bodkin? Who would these fardels bear,To grunt and sweat under a weary life,But that the dread of something after death—The undiscover'd country, from whose bournNo traveller returns—puzzles the will,And makes us rather bear those ills we haveThan fly to others that we know not of?Thus conscience does make cowards of us all;And thus the native hue of resolutionIs sicklied o'er with the pale cast of thought,And enterprises of great pith and momentWith this regard their currents turn awry,And lose the name of action."""# 去掉换行符,并将文本转换为小写text = text.replace('\n', ' ').lower()2 创建N-gram模型接下来,将创建一个N-gram模型,该模型可以接受一个文本字符串,并将其分割成n-gram序列。
def create_ngram_model(text, n): words = text.split() # 将文本分割成单词 ngrams = [] # 用于存储n-grams的列表 for i in range(len(words) - n + 1): ngram = ' '.join(words[i:i + n]) # 创建一个n-gram ngrams.append(ngram) return ngramsn = 2 # 选择2-gram模型ngram_model = create_ngram_model(text, n)# 打印前10个2-gramsprint(ngram_model[:10])在上述示例中,定义了一个create_ngram_model函数,该函数接受文本和n值作为参数,并返回n-gram的列表。选择了2-gram模型(bigram),并打印了前10个2-grams。
3 生成文本有了N-gram模型后,可以使用它来生成新的文本。生成文本的方法是随机选择一个n-gram作为起始点,然后根据模型中的n-gram频率来选择接下来的n-gram,依此类推,直到生成所需长度的文本。
import randomdef generate_text(ngram_model, n, length=50): generated_text = random.choice(ngram_model) # 随机选择一个n-gram作为起始点 words = generated_text.split() while len(words) < length: possible_next_ngrams = [ngram for ngram in ngram_model if ' '.join(words[-n + 1:]) in ngram] if not possible_next_ngrams: break next_ngram = random.choice(possible_next_ngrams) words.extend(next_ngram.split()) generated_text = ' '.join(words) return generated_textgenerated_text = generate_text(ngram_model, n, length=100)print(generated_text)在上述示例中,定义了一个generate_text函数,该函数接受N-gram模型、n值和所需生成文本的长度作为参数。它从模型中随机选择一个n-gram作为起始点,并根据模型中的n-gram频率选择接下来的n-gram,直到生成指定长度的文本。
改进N-gram模型虽然前面的示例中的N-gram模型能够生成文本,但它还有一些局限性。例如,它只考虑了相邻的n-gram,而没有考虑到更远的依赖关系。为了改进模型,可以考虑以下几种方法:
1 增加n-gram的大小通过增加n-gram的大小(如3-gram或4-gram),模型可以捕捉更长范围的依赖关系,生成更具连贯性的文本。但需要注意,增加n-gram的大小也会增加模型的复杂度和数据需求。
# 增加n-gram的大小为3n = 3ngram_model = create_ngram_model(text, n)2 使用更多的训练数据模型的性能通常取决于训练数据的质量和数量。如果有更多的文本数据可用,可以使用更多的训练数据来训练模型,以提高其性能。
3 使用更高级的文本生成技术N-gram模型是一种基本的文本生成技术,但在实际应用中可能需要更高级的方法,如循环神经网络(RNN)或变换器(Transformer)等。这些模型可以学习更复杂的语言结构,生成更具语法和语义的文本。
4 改进文本生成算法改进文本生成算法可以使生成的文本更具连贯性和多样性。一种常见的方法是使用温度(temperature)参数来调整生成的文本多样性,较高的温度会生成更多的随机性,而较低的温度会生成更加确定性的文本。
def generate_text_with_temperature(ngram_model, n, length=50, temperature=1.0): generated_text = random.choice(ngram_model) words = generated_text.split() while len(words) < length: possible_next_ngrams = [ngram for ngram in ngram_model if ' '.join(words[-n + 1:]) in ngram] if not possible_next_ngrams: break # 根据温度参数调整选择下一个n-gram的随机性 next_ngram = random.choices(possible_next_ngrams, weights=[1.0 / temperature] * len(possible_next_ngrams))[0] words.extend(next_ngram.split()) generated_text = ' '.join(words) return generated_text# 使用温度参数为0.5生成文本generated_text = generate_text_with_temperature(ngram_model, n, length=100, temperature=0.5)总结本文介绍了N-gram文本生成的基本原理和实现方法,并提供了示例代码来演示如何创建N-gram模型以及生成文本。通过改进模型的大小、使用更多的训练数据、采用更高级的技术和改进文本生成算法,可以生成更具连贯性和多样性的文本。
N-gram文本生成是自然语言处理中的一个基础任务,但它也有一些限制,特别是在处理复杂的语言结构和语义时。因此,根据具体任务和需求,可能需要考虑更高级的文本生成方法和模型。希望本文的介绍和示例代码能够更好地理解和应用N-gram文本生成技术,从而在文本生成任务中取得更好的效果。