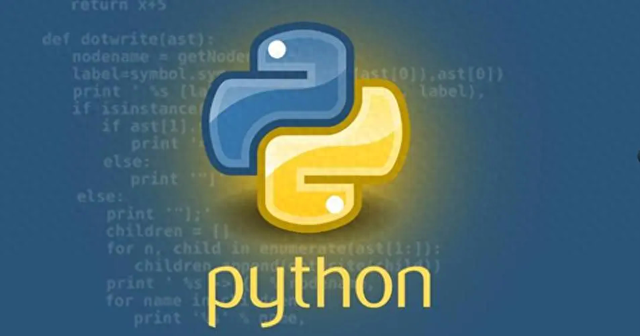
在数据处理中,经常需要找到最接近给定值的元素或数据点。这种需求在科学、工程和统计分析中非常常见。Python 提供了多种方法来实现这种临近匹配。本文将介绍一些常见的方法和示例代码,以帮助大家更好地处理这类问题。
遍历列表最简单的方法是遍历列表,计算每个元素与目标值的差距,并找到最小差距对应的元素。
下面是一个示例:
def find_nearest_element(arr, target): nearest = arr[0] min_diff = abs(nearest - target) for element in arr: diff = abs(element - target) if diff < min_diff: min_diff = diff nearest = element return nearest这个函数 find_nearest_element 接受一个列表 arr 和一个目标值 target,然后遍历列表中的元素,找到与目标值最接近的元素并返回。
使用 min 函数和 key 参数Python 的 min 函数可以接受一个可迭代对象和一个 key 参数,用于指定比较元素的方式。可以使用这个函数来实现临近匹配。
def find_nearest_element(arr, target): return min(arr, key=lambda x: abs(x - target))这个函数使用 lambda 表达式作为 key 参数,它会计算每个元素与目标值的差距,并返回差距最小的元素。
使用 NumPy如果处理的是大型数据集或多维数组,NumPy 是一个强大的工具。它提供了高效的数组操作,包括临近匹配。
import numpy as npdef find_nearest_element(arr, target): arr = np.array(arr) idx = np.abs(arr - target).argmin() return arr[idx]这个函数首先将列表转换为 NumPy 数组,然后使用 np.abs 计算绝对差距,并使用 argmin 找到最小差距对应的索引。
使用二分查找如果列表是有序的,可以使用二分查找来更加高效地找到最接近的元素。
def binary_search_nearest(arr, target): left, right = 0, len(arr) - 1 nearest = None while left <= right: mid = (left + right) // 2 if arr[mid] == target: return arr[mid] elif arr[mid] < target: left = mid + 1 else: right = mid - 1 if nearest is None or abs(arr[mid] - target) < abs(nearest - target): nearest = arr[mid] return nearest这个函数 binary_search_nearest 使用二分查找算法,在有序列表中找到最接近的元素。它不断地将查找范围缩小,同时记录最接近的元素。
临近匹配范围有时候,不仅仅需要找到最接近的元素,还需要找到在一定范围内的所有元素。
下面是一个示例函数,用于找到在指定范围内的所有临近元素:
def find_elements_in_range(arr, target, radius): result = [] for element in arr: if abs(element - target) <= radius: result.append(element) return result这个函数接受一个列表 arr、目标值 target 和一个半径 radius,然后遍历列表中的元素,将在指定范围内的元素添加到结果列表中。
使用二分查找和二叉搜索树如果数据集非常大,而且需要频繁进行临近匹配,可以使用二叉搜索树(BST)来加速匹配过程。
下面是一个示例函数,使用 Python 的 bisect 模块实现了基于二分查找的二叉搜索树:
import bisectclass BST: def __init__(self): self.data = [] def insert(self, value): bisect.insort(self.data, value) def find_nearest(self, target): index = bisect.bisect_left(self.data, target) if index == 0: return self.data[0] if index == len(self.data): return self.data[-1] left = self.data[index - 1] right = self.data[index] if abs(left - target) < abs(right - target): return left else: return right这个示例中,创建了一个 BST 类,使用 bisect 模块中的函数来插入和查找元素。这种方法适用于需要频繁进行临近匹配的场景。
示例代码# 示例列表data = [1, 3, 5, 7, 9, 11, 13]# 目标值和半径target = 6radius = 2# 使用各种方法找到最接近的元素或范围内的元素result4 = find_elements_in_range(data, target, radius)bst = BST()for element in data: bst.insert(element)result5 = bst.find_nearest(target)print("临近匹配范围方法:", result4)print("二叉搜索树方法:", result5)以上示例演示了如何使用新的方法找到最接近目标值的元素或指定范围内的元素。根据你的需求,选择合适的方法来处理不同的临近匹配问题,以提高代码的效率和可维护性。
总结在数据处理中,临近匹配是一个常见的问题,需要找到最接近给定值的元素或在指定范围内的元素。本文介绍了更多的方法,包括临近匹配范围和使用二叉搜索树,以应对不同的场景和需求。希望这些示例代码能够帮助大家更好地理解和应用临近匹配的概念,以及如何在实际项目中处理这类问题。