TinyLlama项目旨在在3万亿tokens上进行预训练,构建一个拥有11亿参数的Llama模型。经过精心优化,我们"仅"需16块A100-40G的GPU,便可在90天内完成这个任务。训练已于2023-09-01开始。
项目地址:
https://github.com/jzhang38/TinyLlama/
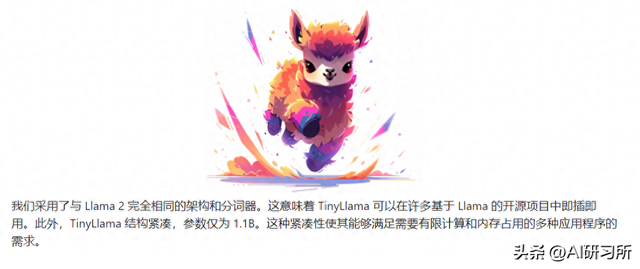
采用了与Llama 2完全相同的架构和分词器。这意味着TinyLlama可以在许多基于Llama的开源项目中即插即用。此外,TinyLlama只有1.1B的参数,体积小巧,适用于需要限制计算和内存占用的多种应用。
发布时间表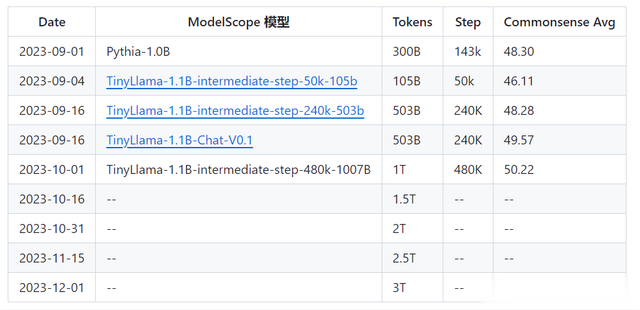
需要注意的是,由于我们的现在模型还处于训练初期,学习率并没有完全稳定下来,为了更好的体验我们的模型,您可以下载我们 聊天模型 或者通过 chat demo 来尝试我们的模型。
潜在场景小型但强大的语言模型对许多应用都很有用。以下是一些潜在的场景:
帮助对大型模型进行speculative decoding。在边缘装置上运行,比如离线的实时机器翻译 (TinyLlama的4比特量化版本的模型权重只需要550MB的内存)。在游戏中实现实时对话生成(因为还得给游戏本身留显存所以模型要小)。此外,我们的代码可以给初学者做一个入门预训练的简洁参考。如果你要训练50亿以下参数的语言模型, 你其实不需要Megatron-LM。
训练细节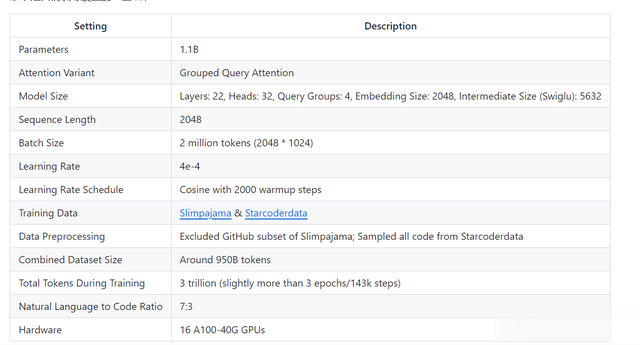
我们的代码库支持以下特性:
multi-gpu and multi-node distributed training with FSDP.flash attention 2.fused layernorm.fused swiglu.fused cross entropy loss .fused rotary positional embedding.Credit: flash attention 2, fused layernorm, fused cross entropy loss, and fused rotary positional embedding are from the FlashAttention repo. Fused swiglu is from xformers.
有了这些优化, 我们可以达到24k tokens/秒/A100的训练速度,也就是56%的MFU(在A100-80G上的MFU会更高)。这个速度可以让你可以在8个A100上用32小时训练一个chinchilla-optimial的模型(11亿参数,220亿token)。
这些优化也大大减少了显存占用, 我们可以把11亿参数的模型塞入40GB的GPU里面还能同时维持16k tokens的per-gpu batch size。只需要把batch size改小一点, 你就可以在RTX 3090/4090上面训练TinyLlama。 下面是我们的代码库与Pythia和MPT的训练速度的比较。
Model
A100 GPU hours taken on 300B tokens
TinyLlama-1.1B
3456
Pythia-1.0B
4830
MPT-1.3B
7920
Pythia的数字来自他们的论文。MPT的数字来自这里,作者说MPT-1.3B"was trained on 440 A100-40GBs for about half a day" on 200B tokens。
TinyLlama是一个相对较小的模型, 同时我们用了GQA, 这意味着它在推理期间也很快。以下是我们测量的一些推理速度:
Framework
Device
Settings
Throughput (tokens/sec)
Llama.cpp
Mac M2 16GB RAM
batch_size=1; 4-bit inference
71.8
vLLM
A40 GPU
batch_size=100, n=10
7094.5
预训练 TinyLlama已安装 CUDA 11.8
安装Pytorchpip install --index-url https://download.pytorch.org/whl/nightly/cu118 --pre 'torch>=2.1.0dev'源构建 XFormers注意:截至 2023 年 9 月 2 日,xformers 不提供 torch 2.1 的预构建二进制文件。您必须从源代码构建它。
pip uninstall ninja -y && pip install ninja -Upip install -v -U git+https://github.com/facebookresearch/xformers.git@main#egg=xformers安装 Flash-Attention 2git clone https://github.com/Dao-AILab/flash-attentioncd flash-attentionpython setup.py installcd csrc/rotary && pip install .cd ../layer_norm && pip install .cd ../xentropy && pip install .cd ../.. && rm -rf flash-attention安装依赖pip install -r requirements.txt tokenizers sentencepiece安装其他依赖项。构建 xformers/flash-attention 可能需要 >= 5 分钟。如果进程似乎停滞或终端打印出许多警告,请不要担心。
数据准备下载数据集将 Slimpajama 和 Starcoderdata 数据集下载到您选择的目录。
cd /path/to/datasetgit lfs installgit clone https://huggingface.co/datasets/cerebras/SlimPajama-627Bgit clone https://huggingface.co/datasets/bigcode/starcoderdataSlimPajama 数据集占用 893GB 磁盘空间,starcoderdata 占用 290GB
标记数据python scripts/prepare_starcoder.py --source_path /path/to/starcoderdata/ --tokenizer_path data/llama --destination_path data/slim_star_combined --split train --percentage 1.0python scripts/prepare_slimpajama.py --source_path /path/to/SlimPajama --tokenizer_path data/llama --destination_path data/slim_star_combined --split validation --percentage 1.0python scripts/prepare_slimpajama.py --source_path /path/to/SlimPajama --tokenizer_path data/llama --destination_path data/slim_star_combined --split train --percentage 1.0处理后的数据将占用1.8T存储空间
预训练如果您的设置包含两个节点,每个节点有 8 个 GPU,您可以使用以下命令启动预训练:
节点1:
lightning run model \ --node-rank=0 \ --main-address=172.16.101.5 \ --accelerator=cuda \ --devices=8 \ --num-nodes=2 \ pretrain/tinyllama.py --devices 8 --train_data_dir data/slim_star --val_data_dir data/slim_star节点 2:
lightning run model \ --node-rank=1 \ --main-address=172.16.101.5 \ --accelerator=cuda \ --devices=8 \ --num-nodes=2 \ pretrain/tinyllama.py --devices 8 --train_data_dir data/slim_star --val_data_dir data/slim_star