简介
通义千问系列模型为阿里云研发的大语言模型。千问模型基于 Transformer 架构,在超大规模的预训练数据上进行训练得到。预训练数据类型多样,覆盖广泛,包括大量网络文本、专业书籍、代码等。同时,在预训练模型的基础之上,使用对齐机制打造了模型的 chat 版本。其中千问-1.8B 是 18 亿参数规模的模型,千问-7B 是 70 亿参数规模的模型,千问-14B 是 140 亿参数规模的模型,千问-72B 是 720 亿参数规模的模型。
Qwen1.5
Qwen1.5 是 Qwen 开源系列的下一个版本。与之前的版本相比,Qwen1.5 显著提升了聊天模型与人类偏好的一致性,改善了它们的多语言能力,并具备了强大的链接外部系统能力。DashScope 上提供 API 服务的是新版本 qwen 模型的 chat 版本,在 chat 能力上大幅提升,即便在英文的 MT-Bench 上,Qwen1.5-Chat 系列也取得了优秀的性能。
Qwen2
Qwen2 参数范围包括 0.5B 到 72B,包括 MOE 模型。Qwen2 在一系列针对语言理解、语言生成、多语言能力、编码、数学、推理等的基准测试中总体上超越了大多数开源模型,并表现出与专有模型的竞争力。Qwen2 增⼤了上下⽂⻓度⽀持,最⾼达到 128K tokens(Qwen2-72B-Instruct),能够处理大量输入
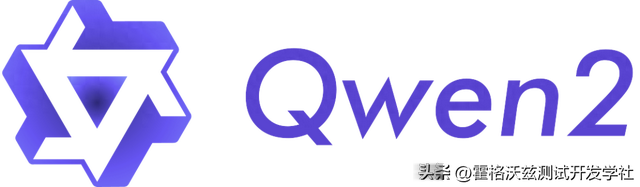
千问 2 性能
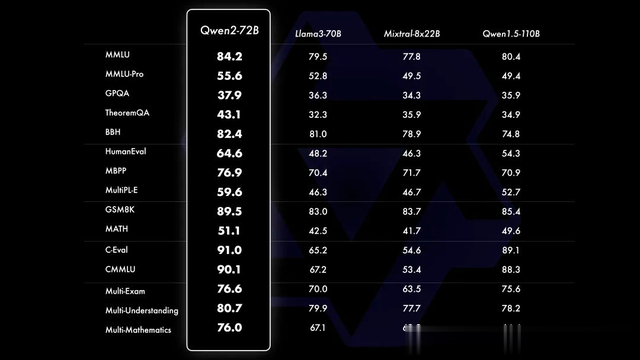
文生文本地部署 ollama
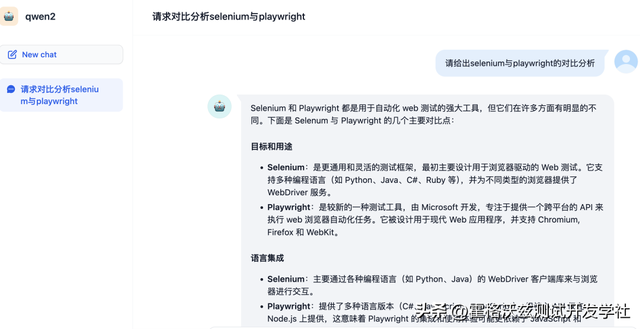
Qwen2-72B-Instruct-demo 在线体验
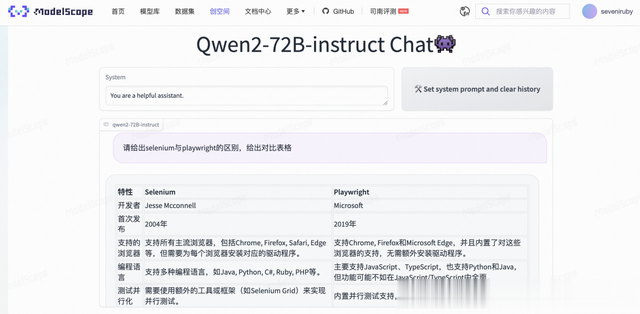
Qwen2-VL ModelScope
Qwen2-VL 可以处理任意图像分辨率,将它们映射到动态数量的视觉标记中,提供更接近人类的视觉处理体验
Qwen2-VL 模型特点读懂不同分辨率和不同长宽比的图片:Qwen2-VL 在 MathVista、DocVQA、RealWorldQA、MTVQA 等视觉理解基准测试中取得了全球领先的表现。理解 20 分钟以上的长视频:Qwen2-VL 可理解长视频,并将其用于基于视频的问答、对话和内容创作等应用中。能够操作手机和机器人的视觉智能体:借助复杂推理和决策的能力,Qwen2-VL 可集成到手机、机器人等设备,根据视觉环境和文字指令进行自动操作。多语言支持:为了服务全球用户,除英语和中文外,Qwen2-VL 现在还支持理解图像中的多语言文本,包括大多数欧洲语言、日语、韩语、阿拉伯语、越南语等。本地部署示例
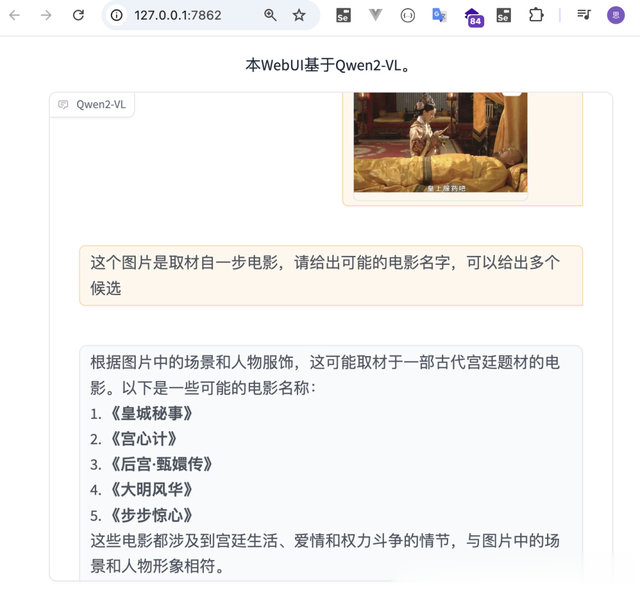
本地处理视频分析
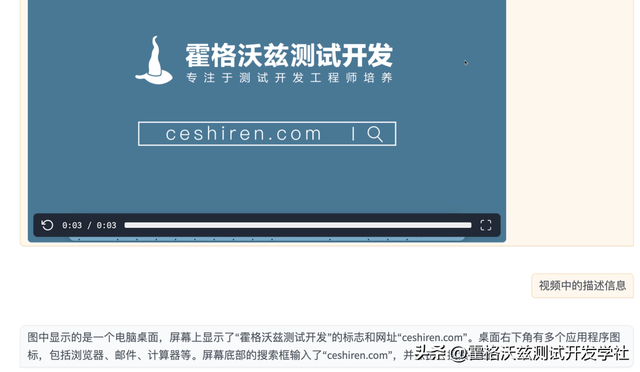
Qwen2-VL ModelScope 在线体验
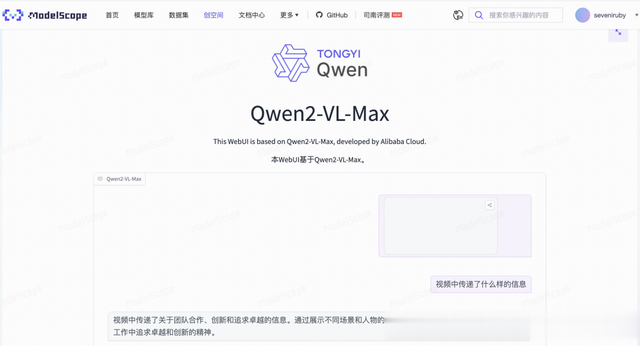
langchain 调用阿里云 apifrom langchain_community.chat_models import ChatTongyifrom langchain_core.messages import HumanMessagechatLLM = ChatTongyi(model_name="qwen-vl-max")image_message = { "image": "https://lilianweng.github.io/posts/2023-06-23-agent/agent-overview.png",}text_message = { "text": "summarize this picture",}message = HumanMessage(content=[text_message, image_message])chatLLM.invoke([message])token 消耗统计content=[{'text': '图中是一位身穿黄色衣服的女子站在床边喂一个男人喝药。女人身穿一身黄色旗袍,上面绣着精美的花纹。男人躺在床上似乎很虚弱的样子。'}] response_metadata={'model_name': 'qwen-vl-max', 'finish_reason': 'stop', 'request_id': '777814e2-873c-93c8-a280-eea5e91f59f1', 'token_usage': {'input_tokens': 335, 'output_tokens': 39, 'image_tokens': 299}} id='run-7708852a-7069-4940-9b25-9bcda0e99e10-0'代码调用 transformers + modelscopefrom PIL import Imageimport requestsimport torchfrom torchvision import iofrom typing import Dictfrom transformers import Qwen2VLForConditionalGeneration, AutoTokenizer, AutoProcessorfrom modelscope import snapshot_downloadfrom utils import debugmodel_dir = snapshot_download("qwen/Qwen2-VL-7B-Instruct")# Load the model in half-precision on the available device(s)model = Qwen2VLForConditionalGeneration.from_pretrained( model_dir, torch_dtype="auto", device_map="auto")processor = AutoProcessor.from_pretrained(model_dir)def test_image(): # Image url = "https://qianwen-res.oss-cn-beijing.aliyuncs.com/Qwen-VL/assets/demo.jpeg" image = Image.open(requests.get(url, stream=True).raw) conversation = [ { "role": "user", "content": [ { "type": "image", }, {"type": "text", "text": "Describe this image."}, ], } ] # Preprocess the inputs text_prompt = processor.apply_chat_template(conversation, add_generation_prompt=True) # Excepted output: '<|im_start|>system\nYou are a helpful assistant.<|im_end|>\n<|im_start|>user\n<|vision_start|><|image_pad|><|vision_end|>Describe this image.<|im_end|>\n<|im_start|>assistant\n' inputs = processor( text=[text_prompt], images=[image], padding=True, return_tensors="pt" ) inputs = inputs.to("cuda") # Inference: Generation of the output output_ids = model.generate(**inputs, max_new_tokens=128) generated_ids = [ output_ids[len(input_ids):] for input_ids, output_ids in zip(inputs.input_ids, output_ids) ] output_text = processor.batch_decode( generated_ids, skip_special_tokens=True, clean_up_tokenization_spaces=True ) debug(output_text)总结功能相对齐全,文本、音频、图片、视频都比较开放在线服务完善 阿里云、魔搭、海外平台集成开放性高,开源,可私有部署